My name is Vatsal and I’m currently pursuing my MBA at the University of Washington. Prior to this, I spent 4 years helping organizations with their data engineering and business intelligence needs, enabling companies to leverage their data assets to drive business value. I worked on enterprise data projects in the Middle East and Australia, primarily within the finance and telecom industry verticals. While the domains were different, the data challenges were the same. I want to share some of the most vexing yet common obstacles that I encountered during this period.
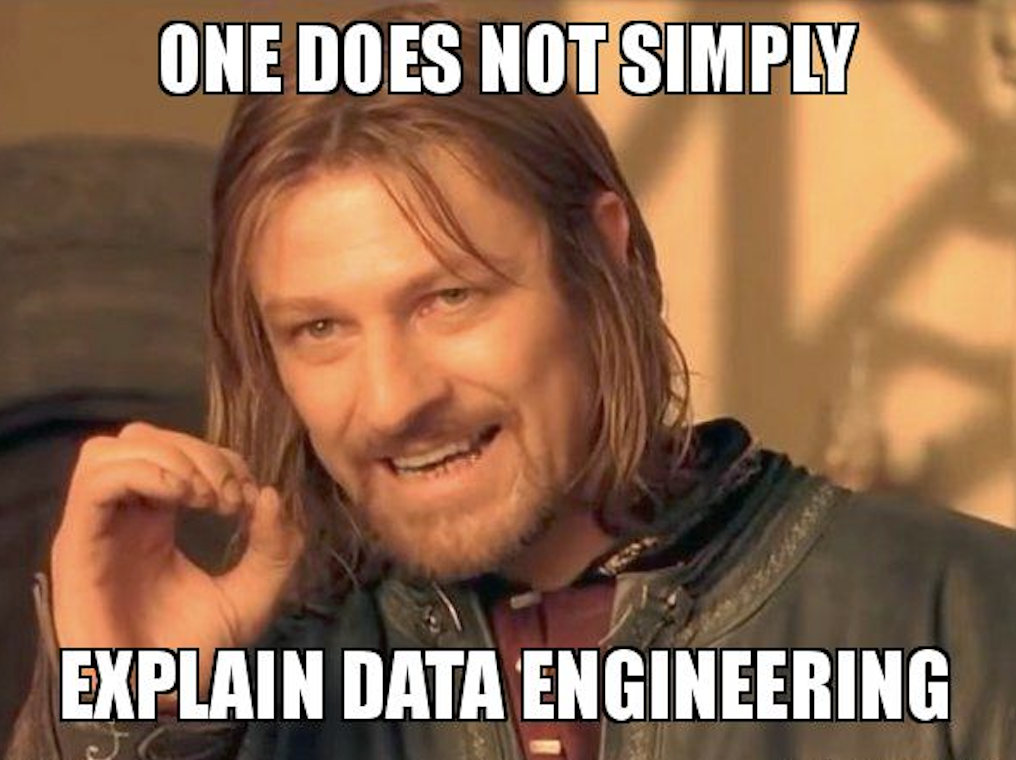
Challenge 1: Data Ingestion — A Maze of Sources and Formats
Imagine traversing a maze where each doorway leads to a data source in a different format — some structured, some chaotic, and some guarded by external gatekeepers. Identifying the right sources, then wrangling them into a unified flow, is the data engineer's Everest. This involves navigating hurdles around identifying relevant data sources, gaining access through proper stakeholders, handling varied data formats like nested JSONs, flat files, databases, streaming data, etc. and collaborating with external data providers.
Collaboration and documentation plays a crucial role in being the source of truth in large scale data transformation and reporting projects. I recall working with a client where they were transitioning from one CRM to another, with both being operational during that transition period. As a data engineer helping them build a reporting platform simultaneously, it was a big challenge to understand what source to look at for what information, the cut off periods and how it impacts the long-term vision and data accuracy of this new reporting platform. Tackling that required intense collaboration between various business and technology teams with documentation playing a key role in continuity and success of the project.
Challenge 2: Data Silos — Bridging the Gap
Organizations often resemble archipelagos of data silos, each one hoarding its own metrics and truths within each business function. This fragmentation leads to confusion, duplication, and ultimately, poor decision-making. This is a key consequence of uncoordinated data ingestion.
A challenge that I faced with a financial industry client that may resonate with my fellow data engineers is seeing a landscape where each department has its own data warehouse, with its own staging, conformed and semantic layers. Not only is there duplication of development effort but also of data. This coupled with redundancy in reporting metrics, as well as the possibility of differences in business logic to arrive at the same metric, has led to organizations to often make decisions in departmental silos with conflicting outcomes in their reporting. Additionally, there was also lack of trust in the data. The time spent in navigating those discussions on a weekly, monthly, or quarterly basis could be better utilized in leveraging the data for more innovative purposes to drive business value.
Challenge 3: Source of Truth — Elevating Business Context
Imagine building a business intelligence dashboard in a data minefield. Each data point could be a potential source of confusion, with conflicting values and conflicting claims of truth. As data unification happens, new questions around "source of truth" emerge. For instance, identifying the right revenue metric from multiple tables to drive executive dashboards. Navigating this requires meticulous data management, documentation, and collaboration to determine authoritative sources. It requires cross collaboration between various stakeholders and teams, sometimes requiring them to come to a consensus so that the organization can have a unified approach and vision.
Institutional knowledge from data owners plays a key role here. Engineering teams can enable self-service analytics only when the underlying data is trusted and consistent. While I’ve had the benefit of having a great working relationship with some of the data owners that allowed me to expedite these discussions, that is not always possible or feasible. Getting this right may require changes in upstream processes and systems with a focus on collaboration between the right stakeholders to document the truth and how it translates to the technical counterparts working with the actual data.
Challenge 4: Changing Management — Overcoming Resistance
Moving from clunky legacy systems to modern cloud platforms can feel like sailing into uncharted waters for some users. The fear of the unknown can breed resistance, slowing down the data transformation process. The journey towards modernized data stacks often requires convincing business users to shift existing habits - whether Excel spreadsheets or legacy business intelligence tools. Migrating from these entrenched approaches to newer self-service platforms like PowerBI or Tableau fuel apprehensions around loss of control or skill gaps.
In my personal experience, it has always been a challenge to transition business users from Excel to PowerBI or similar platforms. What’s been a larger challenge has been working with them to help define the underlying data needs as well as designing their new dashboards without them having an experience of using these new tools that they aren’t as familiar with. What has been lacking has been an intuitive platform for business users to be able to communicate those exact needs to the technical teams to ease out the build and accelerate adoption and transition onto new data platforms.
Change management becomes pivotal even as technological migrations take place. I have learned this first-hand through my transitions focused on automation and making self-service analytics more accessible to business teams. Patience and listening (collaboration) lead to higher user adoption.
Challenge 5: Data Governance — Management and Scaling
As data becomes the central nervous system of an organization, ensuring proper governance becomes paramount. Complex questions emerge around who can view or modify which data. Risks must be evaluated around data breaches, unauthorized modifications and meeting regulatory needs.
Instituting data governance through consensus requires understanding nuances around data sensitivity and organizational structures. Getting user groups aligned on policy priorities based on use cases is an evolutionary process. Starting small while keeping an eye on the big picture helps smooth the transition towards a data-driven culture.
Summary
Across each of the challenges I faced as a data engineer, a common theme emerged: the importance of collaboration and documentation in navigating data initiatives. As a data enthusiast and a business person, I'm excited to see technology solutions that can address this bottleneck and help businesses better leverage their data assets.